Understanding Generative AI: Definition, Examples, and Use Cases
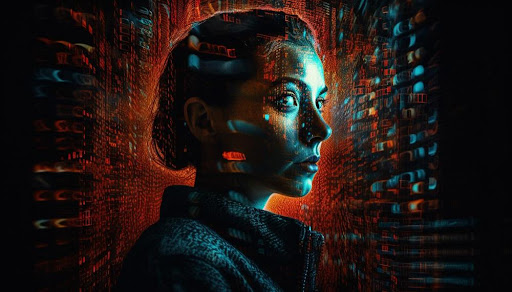
Imagine you’re sketching the basic outline of a cat on a digital canvas. The next moment, you click a button, and, voilà, your simple sketch transforms into a detailed, colorful artwork of a cat.
It looks as if an artist spent hours perfecting it, but it’s all the magic of Generative AI that filled in the gaps and enhanced your basic outline in seconds.
Welcome to a world where Artificial Intelligence doesn’t just analyze but creates. A world where AI takes on the role of an artist, a designer, a musician, or even a writer.
In this blog, we’ll talk about Generative AI in detail and explore various examples, its intriguing uses, and the transformative potential it holds across industries.
What is Generative AI?
Generative AI is a type of artificial intelligence, specifically a subset of machine learning, that is designed to generate new content. It learns from existing data, identifies patterns and features, and then uses that understanding to create new data that resembles the original.
Through generative AI, you can create anything from images, music, and text. The type of data you receive depends on the specific application and the type of data the AI was trained on.
One of the key technologies that supports generative AI is the concept of Generative Adversarial Networks (GANs). Ian Goodfellow and his colleagues introduced GANs in 2014, and they consist of two parts:
- A “generator,” which creates new data instances
- A “discriminator,” which tries to distinguish between real and generated instances.
The two networks are pitted against each other, which leads to continuous improvements in the quality of the generated content.
However, GANs aren’t the only method for building generative models. Other approaches include Variational AutoEncoders (VAEs) and transformer-based models, among others.
There are several potential applications of Generative AI, and they touch almost every industry. Let’s explore these applications in detail.
Top 6 Use Cases and Applications of Generative AI
Here are six significant applications of Generative AI:
Text Generation
Have you ever noticed how your email client suggests responses or how your phone predicts your next word as you type? Those are examples of AI-powered text generation. It is perhaps one of the most familiar applications of generative AI.
OpenAI developed GPT-4 in March 2023, and it’s among the exceptionally powerful text generation models capable of producing human-like text based on the prompts given to it. It can help with:
- Content creation
- Writing assistance
- Language translation
- Time management
- General knowledge and more
Sentiment Analysis
While sentiment analysis typically falls under predictive or descriptive AI, Generative AI models can also contribute significantly.
For instance, AI models can use large amounts of text training data—like reviews or social media comments—to generate responses or insights that replicate human understanding of sentiments. They can also create new data points for further analysis. It can help improve models for medical research, customer service, market research, or public opinion polling.
Image Generation and Enhancement
Generative AI has significantly impacted the way we perceive images. It has the ability to create new pieces of art. A prime example is the AI-generated painting “Portrait of Edmond de Belamy”.
AI can also enhance the quality of low-resolution images. It has opened the doors for creating realistic virtual environments used in video games and simulations. Besides, AI can generate fake yet realistic images, and you can use these images for testing and training other AI systems.
Code Generation
Software development is another field where Generative AI has started to make an impact. Code generation AI can produce code snippets based on natural language descriptions, assist with bug fixing, or even create simple software based on a set of requirements.
Companies like GitHub are using these capabilities to create tools like GitHub Copilot, which assist developers by suggesting lines or blocks of code as they work.
Audio Generation
The ability of Generative AI to produce audio has revolutionized various industries. It can generate new music based on specific styles or artists, create sound effects for movies or video games, or produce synthetic voices for audiobooks or virtual assistants.
OpenAI’s MuseNet is an example of an AI that can generate 4-minute musical compositions with 10 different instruments, spanning a wide range of styles and genres.
Synthetic Data Generation
One of the most important applications of Generative AI is that you can get synthetic data. In areas where it’s costly, time-consuming, or privacy-infringing to collect data, AI can generate synthetic datasets that preserve the statistical properties of the original data without compromising privacy. This is particularly useful in fields like healthcare, finance, or any area where sensitive data is involved.
Generative AI, in its essence, is a powerful creative tool. When you tap into the potential of this technology effectively, it can innovate and transform various sectors. However, like any technology, it should be used responsibly, considering its potential ethical implications.
Conclusion
Generative AI is an exciting frontier that is redefining the boundaries of technology and creativity. From generating human-like text to creating realistic images, enhancing code development, and even simulating unique audio outputs, its real-world applications are as diverse as they are transformative.
As we continue to embrace and refine this technology, the future beckons with even more innovative and intriguing possibilities that surpass our traditional understandings of AI.